How Edge AI Differentiates Between Left Brain and Right Brain AI
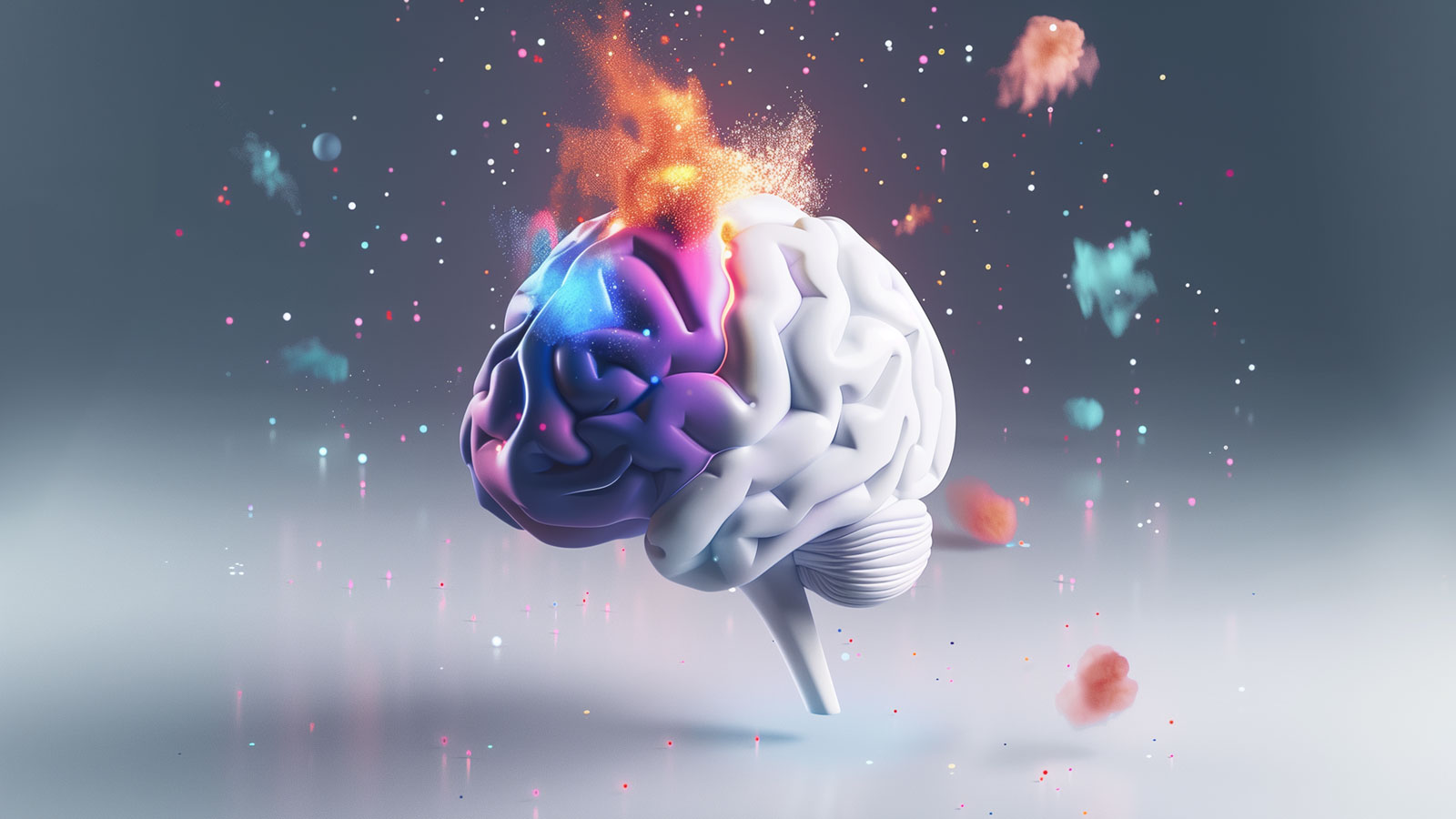
Artificial Intelligence (AI) has evolved to encompass a broad spectrum of capabilities, reflecting both logical (left-brain) and creative (right-brain) functions. Understanding how Edge AI differentiates between these aspects can provide valuable insights into the future of AI applications and their impact on various industries.
This article delves into the distinctions between left-brain and right-brain AI, explores the role of Edge AI in these dynamics, and introduces ContextSDK as a tool that enhances understanding and application of AI in real-world scenarios.
Understanding The TraditionalTraditoinal View of Left Brain vs. Right Brain in AI
Left Brain AI: Logical and Analytical
Traditionally, the left brain is associated with logical thinking, analytical reasoning, and language processing. In the realm of AI and large language models, this translates to systems designed for tasks involving structured data and rule-based processing. Key characteristics of left-brain AI include:
- Data Analysis: AI systems that excel at processing and analysinganalyzing large datasets to identify patterns and insights.
- Pattern Recognition: Algorithms that detect patterns and trends in data, useful for applications like fraud detection and predictive analytics.
- Logical Reasoning: Systems that follow predefined rules and algorithms to solve problems and make decisions.
For more detailed insights into left-brain AI, see Agile Brand Guide’s article on Left Brain vs. Right Brain AI.
Right Brain AI: Creative and Intuitive
The right brain is linked to creativity, intuition, and holistic thinking and in the realm of Edge AI. It focuses more on reflex based intuitive intelligence, which aims to be very fast but not always 100% correct - eg more creative. In AI, this translates to systems that try to mimic human creativity and emotional intelligence. Right-brain AI involves:
- Generative Design: AI that creates new and novel designs, art, or music, showcasing its creative capabilities.
- Natural Language Processing (NLP): Systems that understand and generate human language, enabling applications like chatbots and virtual assistants.
- Intuitive Problem-Solving: AI that can adapt and provide solutions in unstructured or unpredictable environments.
Explore more about right-brain AI in Quora’s discussion on AI’s focus.
Understanding Left and Right Side 'AI Brain' in Coatuе’s AI S-Curve: Evolutionary AI Phases"

The Coatuе Framework for the AI S-Curve provides a different take on the Left v Right side of brain for AI. In the talk by Phillipe Lafont (Founder of Coatue Management) at the EMW Conference, provides a view of the evolutionary phases of AI development, highlighting the stages from foundational infrastructure to advanced physical AI applications in taken. He refers to the left side as the initial technological build out majoritively in Phase 1, focused on analysis of large complex problems and data. In Phase 2, which he refers to as the right side of the brain, the evolutionary focus starts to incorporate more reflex based intuitive intelligence and considered more edge AI at at the device level. Finally evolving into more autonomous applications of AI away from Phase 1 of datacenters and Phase 2 of device level to more stand alone applications like robotics and autonomous agents of Phases 3 and 4.
Phase 1: AI Core Infrastructure
This phase lays the groundwork for AI's development, focusing on the essential hardware and infrastructure required to power AI technologies. It includes:
- GPU (Graphics Processing Units): The engines that accelerate AI computations, making complex tasks manageable.
- Datacenter: The backbone that stores and processes vast amounts of data, enabling AI to function at scale.
- Power: Ensuring sufficient and sustainable energy resources to support AI operations.
- Cloud: Providing scalable and flexible computing resources that allow AI models to be trained, deployed, and accessed globally.
Phase 2: Edge AI
In this phase, AI moves closer to the user, integrating into devices that people use daily. Edge AI signifies a shift from centralized AI systems to more distributed, accessible technologies:
- AI Smartphones: Bringing AI directly into consumers' hands, enabling features like voice assistants, predictive text, and personalized recommendations.
- AI PCs: Enhancing personal computing with AI capabilities, improving productivity, security, and user experiences.
Phase 3: AI Applications
AI's integration into various applications becomes more profound and specialized in this phase, leading to innovations in automation and personal assistance:
- AI Personal Assistants: Virtual assistants that help manage daily tasks, from scheduling to information retrieval, growing increasingly intuitive and capable.
- Co-Pilot: AI systems designed to assist professionals in specific domains, providing real-time support and insights.
- Autonomous Agents: AI-driven systems that can operate independently, making decisions and taking actions without human intervention.
- AI Software SKUs: Specialized AI software solutions tailored to specific industry needs.
- AI Customer Service Agents: AI-powered agents that handle customer inquiries, providing instant, accurate, and personalized responses.
Phase 4+: Physical AI
The most advanced stage, where AI transcends virtual environments and becomes a tangible part of the physical world. This phase includes:
- Self-Driving: Autonomous vehicles that navigate and operate without human drivers, potentially revolutionizing transportation.
- AI Robots / Humanoids: Robots that interact with the physical world, performing tasks ranging from simple assistance to complex, human-like activities.
- Robotic Surgery: Precision surgeries performed by AI-driven robots, reducing the risk of human error and improving outcomes.
- AI Drug Discovery: Accelerating the discovery of new drugs through AI's ability to analyze complex biological data and predict effective compounds.
The S-Curve in this framework represents the gradual yet accelerating pace of AI's progress across these phases. It underscores how AI evolves from foundational technologies to transformative applications that could reshape entire industries. As we move further along this curve, the potential for AI's impact on society continues to expand, making it crucial to understand these phases and prepare for the advancements they herald.
How Edge AI Bridges the Gap Today
Edge AI refers to the deployment of AI algorithms and processing at the edge of the network, closer to the source of data. This approach allows AI systems to operate in real-time and make decisions locally, enhancing their efficiency and responsiveness. Edge AI plays a crucial role in balancing left-brain and right-brain functions:
Combining Analytical and Creative Functions
Edge AI systems are increasingly designed to integrate both analytical (left-brain) and creative (right-brain) functions. This integration allows for more holistic and adaptable AI applications:
- Real-Time Data Processing: Edge AI can process data in real-time, enabling quick responses and decisions based on both analytical insights and creative inputs.
- Adaptive Learning: AI at the edge can learn and adapt based on both structured data and user interactions, providing a balanced approach to problem-solving.
Practical Applications
Edge AI is applied in various industries to enhance both analytical and creative tasks. Examples include:
- Smart Devices: Edge AI in smart home devices can manage energy consumption (analytical) while also responding to user preferences and patterns (creative).
- Healthcare: AI-powered medical devices can analyze patient data (analytical) and provide personalized treatment recommendations based on individual health patterns (creative).
For more on the integration of AI capabilities, read Psychology Today’s article on the left-brain and right-brain dynamics of LLMs.
Enhancing AI with Real-World Context with ContextSDK
As AI technology evolves, tools like ContextSDK offer advanced solutions for enhancing AI capabilities. Here’s how ContextSDK contributes:
Real-World Contextual Awareness
ContextSDK utilizes over 180 mobile signals to provide real-world context about a user’s activity, such as whether they are walking, sitting, or in transit. This contextual data enhances AI’s ability to deliver relevant and timely interactions, blending analytical precision with creative flexibility.
- Personalized Experiences: By understanding the user’s current context, ContextSDK helps tailor interactions to fit their immediate needs and preferences, improving user engagement.
- Optimized Performance: ContextSDK’s real-world analysis supports both logical and creative applications, optimizing performance and user experience.
Privacy and Security
ContextSDK emphasizes user privacy by performing all context analysis on-device. This approach ensures that user data remains secure and private, aligning with modern data protection standards.
- On-Device Processing: All context analysis occurs on the user’s device, avoiding the need for cloud transfers and ensuring data privacy.
Tools for Enhanced AI Integration
ContextSDK provides tools like Context Insights and Context Decision to further enhance AI applications:
- Context Insights: Offers valuable data on user behavior through real-world context, aiding in both analytical and creative AI tasks.
- Context Decision: Identifies ideal moments for user engagement, optimizing both logical responses and creative interactions.
For more information on ContextSDK’s capabilities, visit ContextSDK’s blog on building the infrastructure for context events.
Conclusion
Edge AI is transforming the landscape of artificial intelligence by bridging the gap between left-brain (logical) and right-brain (creative) functions. Understanding this dynamic allows businesses to leverage AI more effectively, combining analytical precision with creative adaptability and better able to leverage the evolutionary phases of AI. Tools like ContextSDK are very much already in Phase 2 of the evloutio and leverage real-world contextual awareness, privacy-focused data processing, and advanced AI tools to the world of mobile apps.
For additional insights into AI’s evolving capabilities and practical applications, explore General Motors' AI initiatives with Google Cloud and the latest research on intent recognition.
By embracing these advancements and tools, businesses can stay ahead in delivering exceptional AI-driven experiences and optimizing their applications.