What Is Biased Attribution?
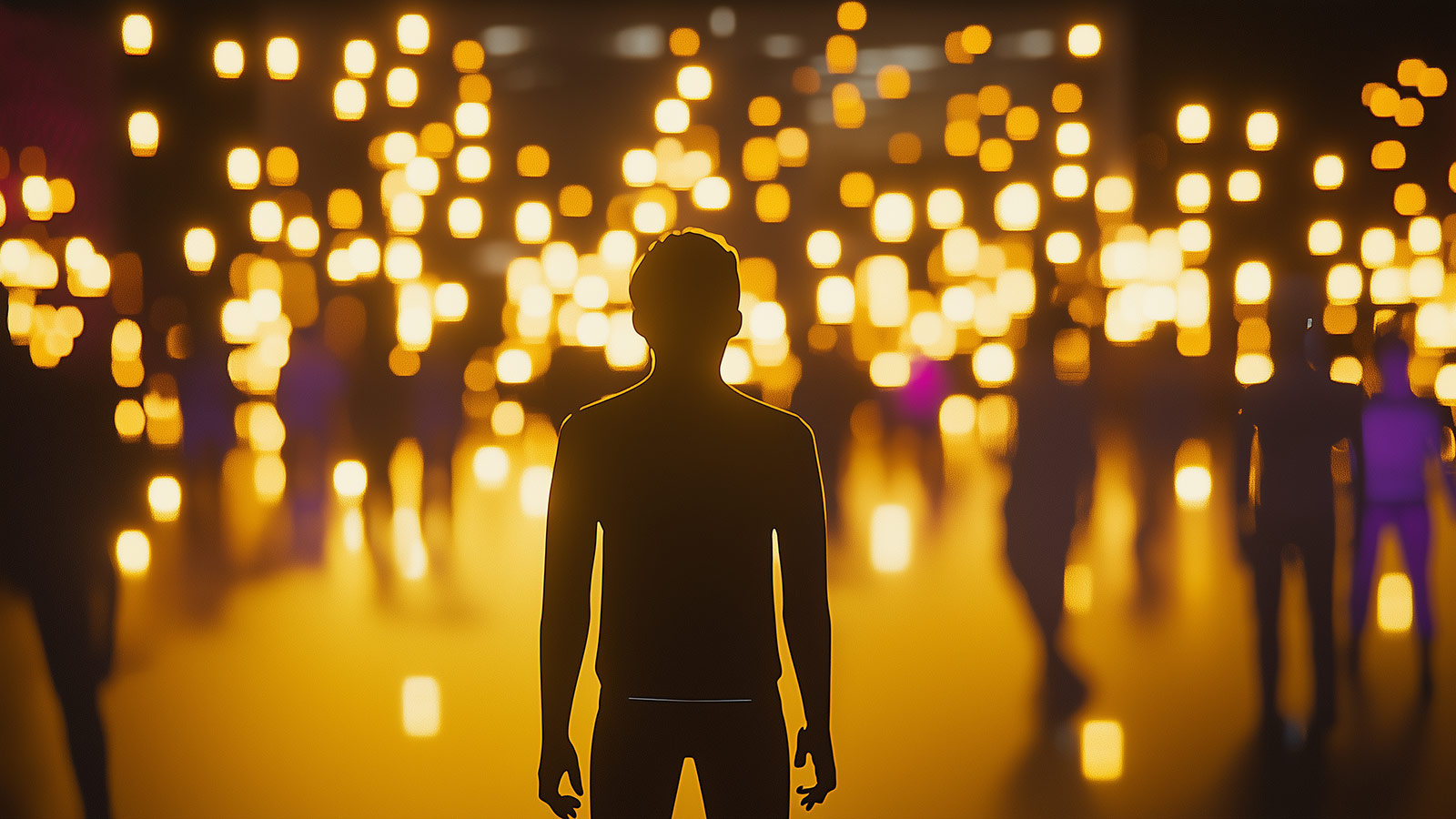
Introduction
Biased attribution refers to the tendency for individuals to systematically distort the reasons or explanations they assign to behaviors or events. In the context of user engagement, product performance, or marketing, biased attribution can affect how data is interpreted, leading to misjudgments and flawed decisions. Understanding these biases is crucial for businesses that rely on data analytics to optimize their strategies. This concept is particularly relevant for mobile apps and digital platforms where user behavior is tracked and analyzed to improve engagement. Attribution bias can cloud the understanding of what drives user actions, potentially leading to misguided optimizations.
The impact of biased attribution extends beyond just decision-making in businesses. In psychology, it influences how people perceive the causes of events in everyday life, such as attributing someone's success to their innate abilities or blaming external circumstances for their failures. This human tendency to favor certain types of explanations over others has been studied extensively. In marketing and user analytics, it can lead to focusing on the wrong metrics, resulting in lost opportunities. Learn more about biases in attribution through this comprehensive guide.
Types of Biased Attribution
There are several types of attribution biases that can distort the interpretation of user behavior data. Some of the most common include:
1. Self-Serving Bias
This occurs when individuals attribute their successes to internal factors (like skill or effort) and their failures to external factors (like bad luck or others' mistakes). In a business context, companies may over-credit successful marketing campaigns to superior strategy while blaming external factors for poor results. This leads to an unbalanced view of performance and can skew decision-making.
2. Fundamental Attribution Error
This bias happens when people overemphasize personal characteristics and underestimate situational factors when explaining others’ behavior. For instance, in a workplace setting, managers might attribute a team member's low productivity to laziness rather than considering external factors like unclear instructions or overburdening workload. The same misjudgment can happen when interpreting user data, such as assuming a lack of interest based on a drop in engagement without accounting for external factors like app downtime or technical issues. This article explores the effect of fundamental attribution error in various contexts.
3. Confirmation Bias
Confirmation bias is the tendency to search for or interpret information in a way that confirms one’s preexisting beliefs. In digital marketing and analytics, this bias can cause businesses to focus only on data that supports their assumptions, disregarding data that contradicts them. This can lead to flawed insights and missteps in strategy. To avoid confirmation bias, it's essential to consider all data sources and viewpoints when analyzing user behavior.
4. Actor-Observer Bias
This bias refers to the tendency to attribute one's own actions to external causes while attributing others' actions to internal causes. For instance, a business may attribute a sudden dip in user engagement to an external factor (e.g., a holiday season) while attributing similar drops in a competitor's engagement to their inferior product or service. Recognizing actor-observer bias can help businesses develop a more nuanced understanding of market trends and user behaviors.
Addressing Biased Attribution in User Analytics
Using Objective Metrics
The key to overcoming biased attribution is to rely on objective, comprehensive data. In user analytics, relying solely on metrics like Daily Active Users (DAU) or Monthly Active Users (MAU) might lead to biased conclusions. It's important to analyze a broad range of metrics and contextual data to get a holistic view of user behavior.
Contextual Data and Mobile Signals
One way to combat biased attribution is by collecting and analyzing data that reflects real-world user behavior. ContextSDK, a leading Edge AI startup, offers a solution by providing mobile apps with insights based on real-world contexts. Instead of focusing solely on surface-level metrics, ContextSDK processes over 200 mobile signals to understand a user’s environment and activity. This allows businesses to accurately determine what’s influencing engagement and performance, reducing the chance of misattributing user behaviors to incorrect causes.
For instance, ContextSDK can detect whether a user is experiencing a quiet morning or is active and on-the-go, allowing for more informed decisions on when to send notifications or prompts. This reduces the chances of relying on biased interpretations and helps tailor engagement strategies to real-world behaviors.
Tools to Reduce Biased Attribution in Analytics
To effectively combat biased attribution, businesses can utilize advanced analytics tools that focus on both quantitative and qualitative data. ContextSDK’s ContextPush is one such tool that provides actionable insights into user behavior by sending push notifications at the perfect moment, ensuring the highest open rate and lowest opt-out rate. These insights are drawn from on-device signals, ensuring that they are accurate and privacy-compliant.
With tools like ContextDecision and ContextPush, businesses can gain a deeper understanding of how and when users engage with their app in various situations. This helps prevent biased conclusions based on incomplete data. Additionally, the ContextDecision tool from ContextSDK leverages over 200 signals to pinpoint the ideal moments for in-app offers, enhancing conversion rates by over 60%. By combining data from multiple touchpoints, these tools allow businesses to develop a more accurate and balanced view of user behavior.
ContextSDK also emphasizes user privacy, with all data processing happening on the user's device. No personal identifiable information (PII) is collected, ensuring that businesses can analyze user behavior without compromising privacy. This privacy-first approach helps maintain user trust while still providing valuable insights.
Real-World Examples of Biased Attribution in Action
Businesses across various industries can fall victim to biased attribution if they don't utilize comprehensive data sources. For example, an e-commerce company might incorrectly assume that a sudden drop in sales is due to a poor marketing campaign. In reality, external factors like a temporary website glitch or economic downturn might be at play. By integrating real-world data and more detailed analytics, businesses can uncover the true causes behind their performance metrics.
Similarly, biased attribution can affect mobile app engagement. Imagine a fitness app that experiences a decline in user activity during certain hours. Without considering real-world context, the app developers might attribute this drop to user disinterest. However, by using tools like ContextSDK, they might discover that users are simply busy commuting or resting, offering a clearer picture of their engagement patterns.
Conclusion: Overcoming Biased Attribution with Comprehensive Insights
Understanding and addressing biased attribution is crucial for businesses looking to make data-driven decisions. By recognizing the types of biases that can distort user analytics, companies can take proactive steps to ensure their strategies are based on accurate data. Tools like ContextSDK can play an essential role in reducing attribution bias by providing a comprehensive view of user behavior, grounded in real-world context. This approach ensures that businesses can enhance engagement without falling victim to the common pitfalls of biased attribution.
Relevant Links:
- ContextSDK
- Attribution Bias in Psychology
- Fundamental Attribution Error
- User Engagement KPIs
- Preventing Bias in Analytics
This balanced approach to user engagement and data analysis helps businesses achieve success while maintaining transparency and user trust.